
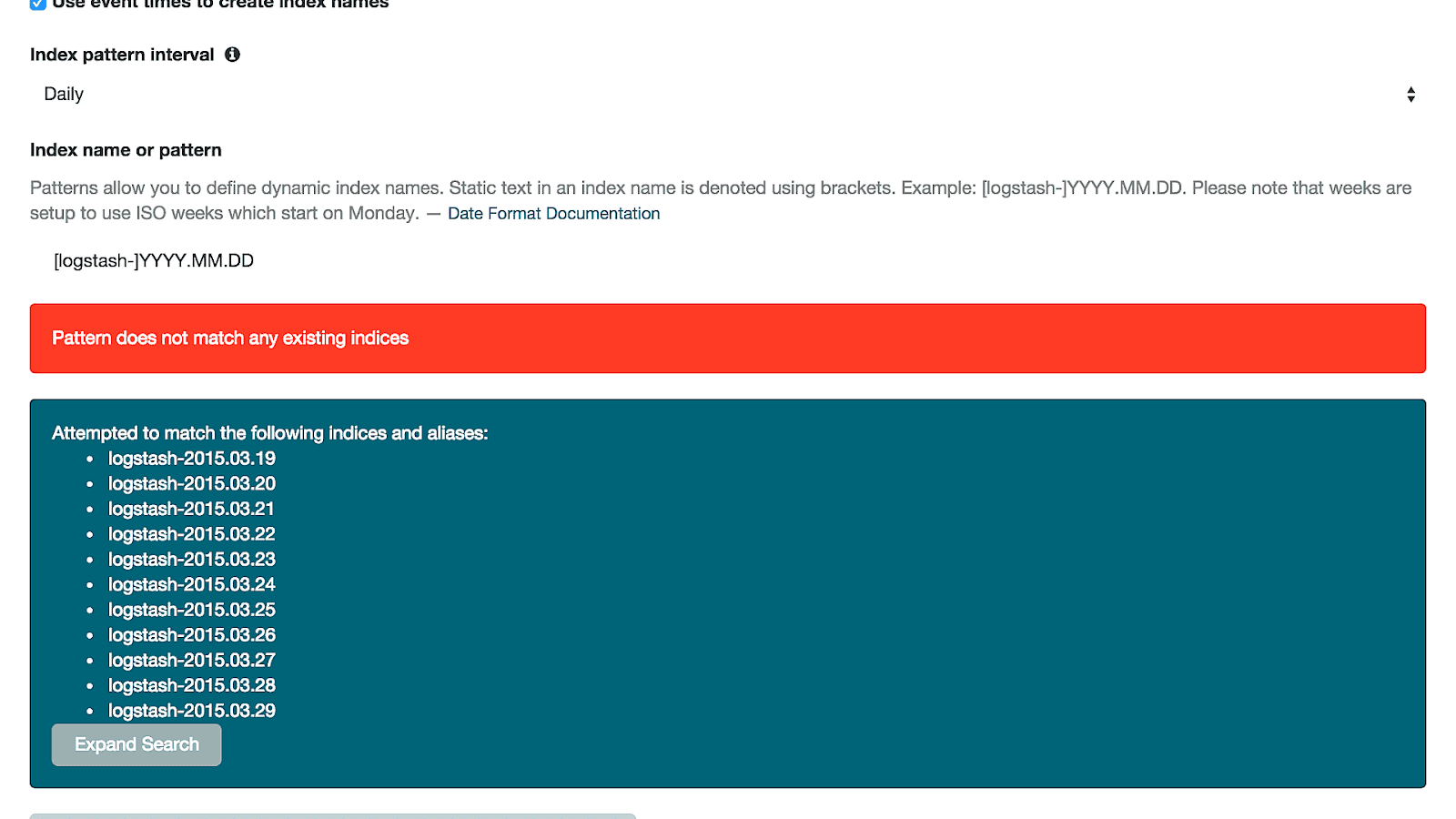
But since these do not scale with increasing data and workload, Elasticsearch is quickly becoming a popular alternative.
#Elasticsearch metabase series
While storing time series based metrics, InfluxDB and Prometheus are some of the popular choices.
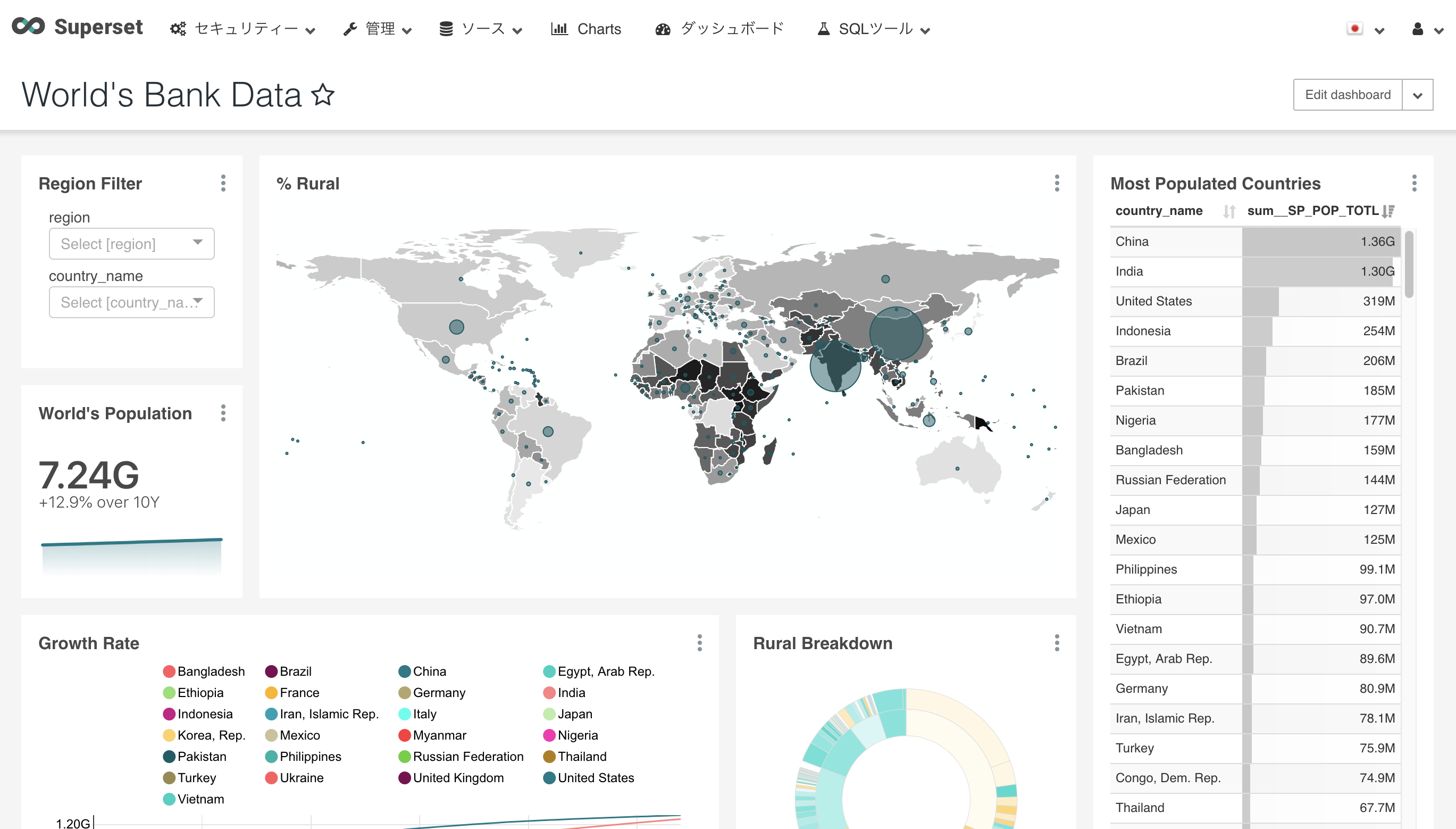
Velocity comprises of following components: Kibana displays the data through dashboards. Custom jobs deployed on the Flink cluster read the events from Kafka, perform the aggregations and store the result in Elasticsearch indices. AWS Lambda functions are used to enrich this event data (by calling the REST APIs of microservices) and enriched events are pushed into Kafka topics. Architectureĭata from individual microservices are currently published to SNS (a lightweight eventing system between microservices). This drove the need for a separate real-time data pipeline and Velocity was born. Non-availability of data on RedShift (in real-time) was not a concern for reporting but was a major change for the real-time dashboards. Hence, instead of DMS jobs (that copy the data in realtime), the data team planned to use the ETL jobs to copy the data to Redshift in batches (with separation of multiple hours).
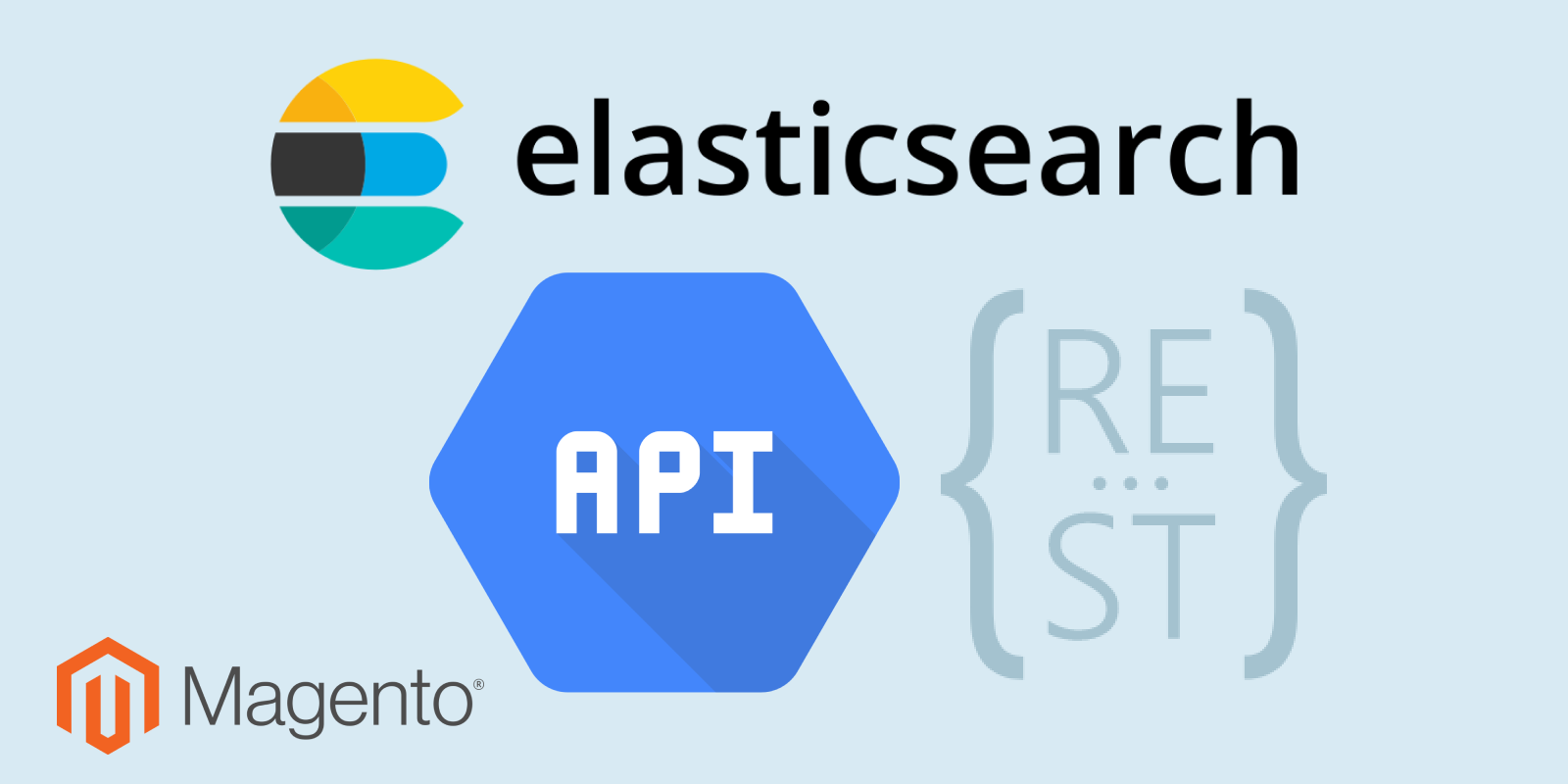
When the data team analysed the root cause, we found that RedShift, like most Data Warehouse systems, is optimised for batch updates, especially when the read traffic is less. This started impacting the performance of the RedShift system, along with a substantial increase in query response time. But with an increase in business, we saw our backend data sources increase rapidly. The data in Redshift is used by the operations team for various purposes like BI Reporting (through PowerBI/Looker), real-time monitoring of business metrics (Metabase dashboard) and ad-hoc queries (Metabase Questions). The DMS jobs copied any edited data over to Redshift in real-time. Given how querying data from multiple sources might slow down our operations, we set up our data platform to copy the data from individual databases to AWS Redshift via DMS jobs. But from Halodoc's operations point-of-view, a single transaction by a user might span across multiple business domains. Business domains like Pharmacy Delivery, Tele Consultations, Appointments, and Insurance have their own microservices. These microservices have access to their data but rely on the APIs to retrieve data from other microservices. Halodoc backend consists of multiple microservices that interact with each other via REST APIs.
